When using different GenAI models, you may encounter biases stemming from unequal representation in the training data. This can manifest as gender biases and a lack of diversity in the subjects depicted in the generated images.
Some examples of bias found when using GenAI image models:
Prompt: engineer
All the images generated show white, male subjects.
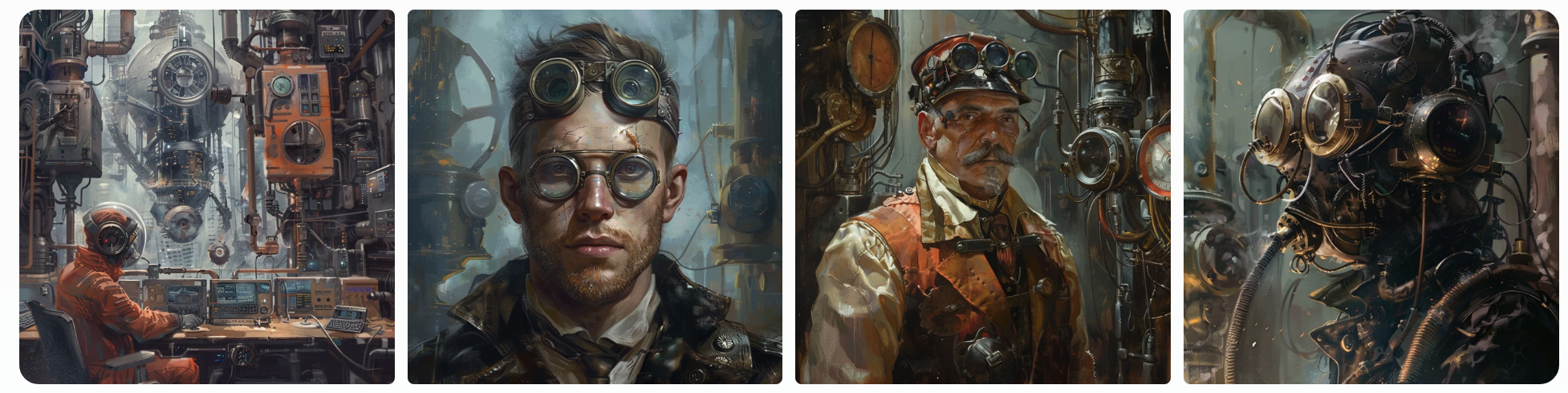
Prompt: nurse
All the images generated show white, female subjects.
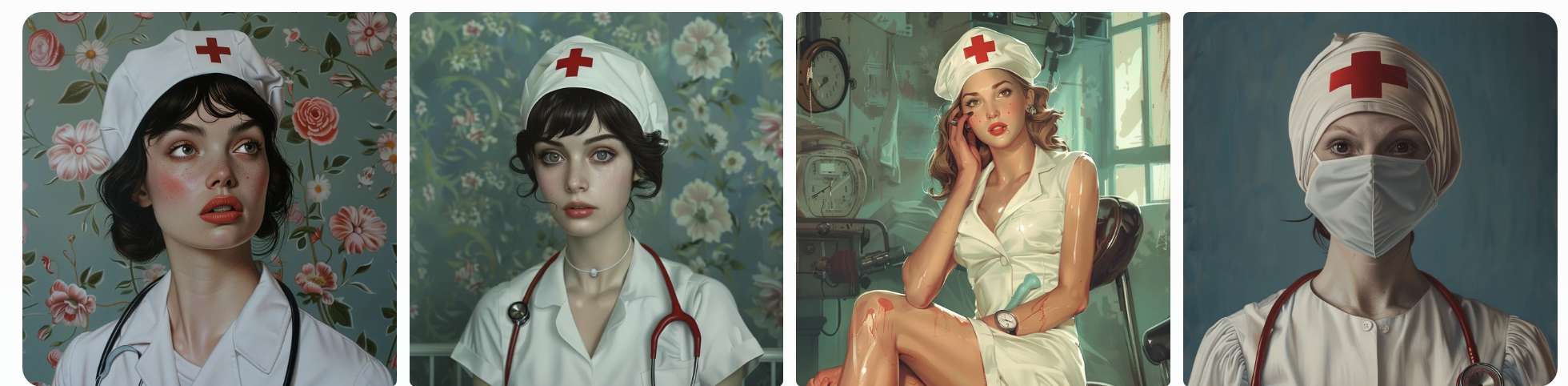
Prompt: doctor
All the images generated show white, male subjects.
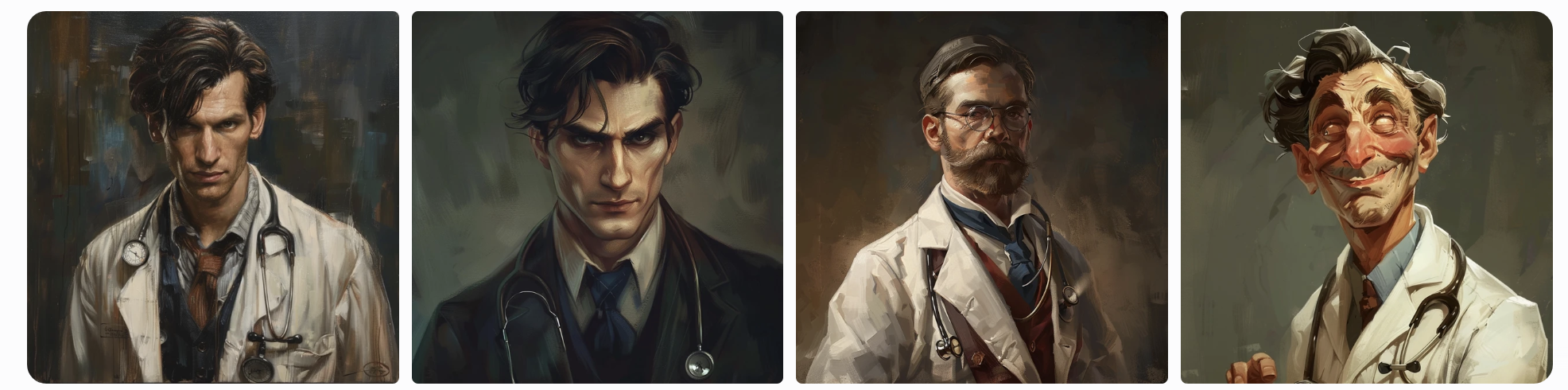
Prompt: firefighter
All the images generated show white, male subjects.
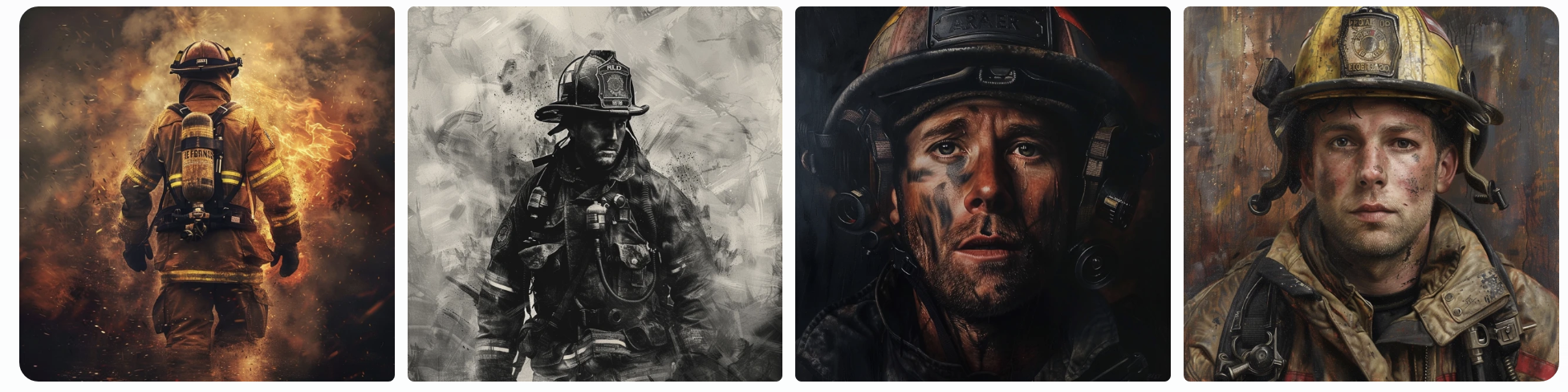
Prompt: police officer
All the images generated show white subjects, with one female and three males.
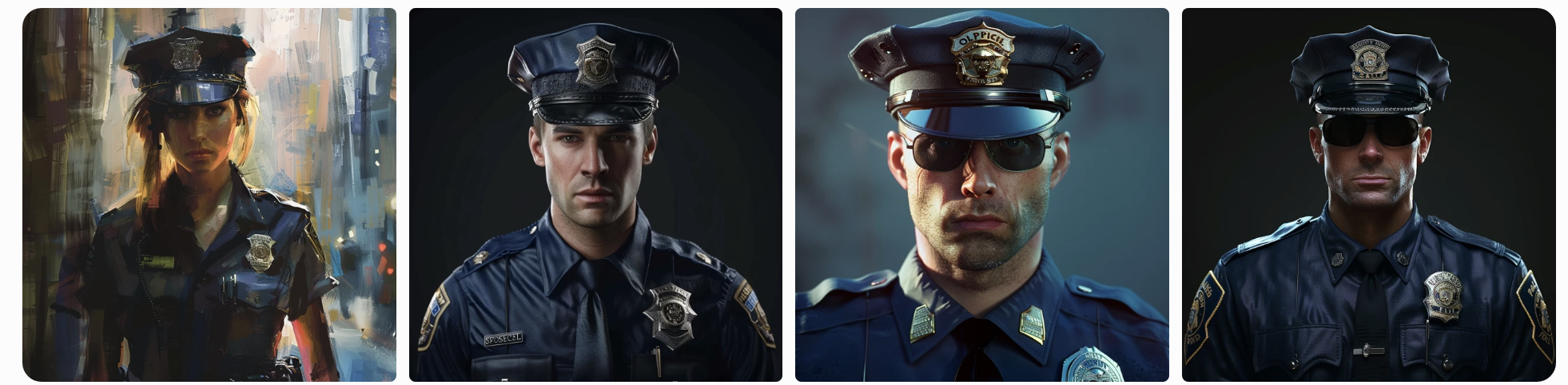
Prompt: scientist
All the images generated show white subjects, with one female and three males.
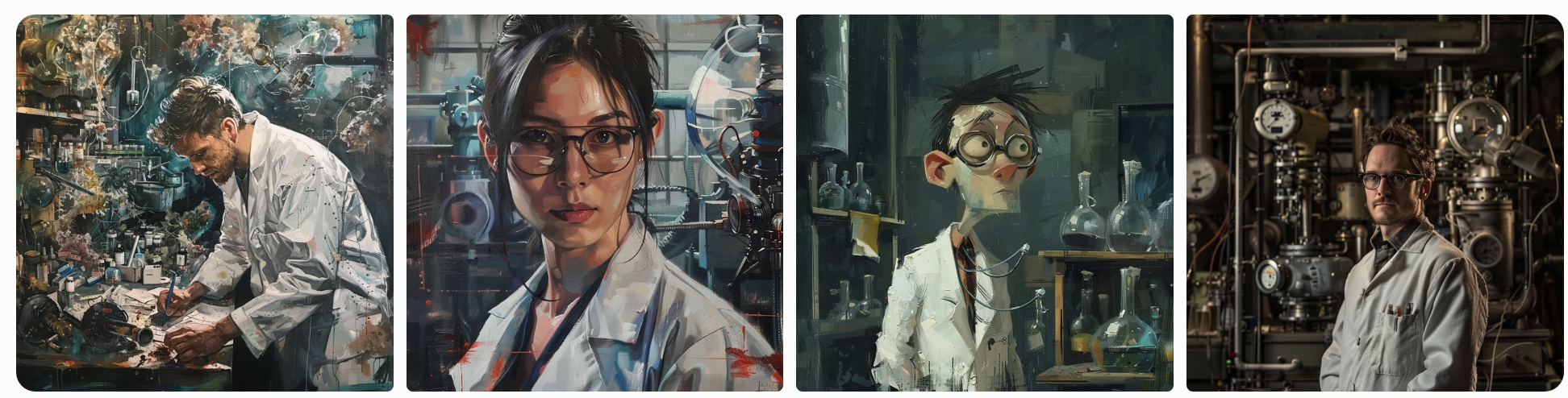
Prompt: parent at home taking care of kids
Three of the images generated show white subjects, one image shows a hispanic mother. There is one male and three female subjects.
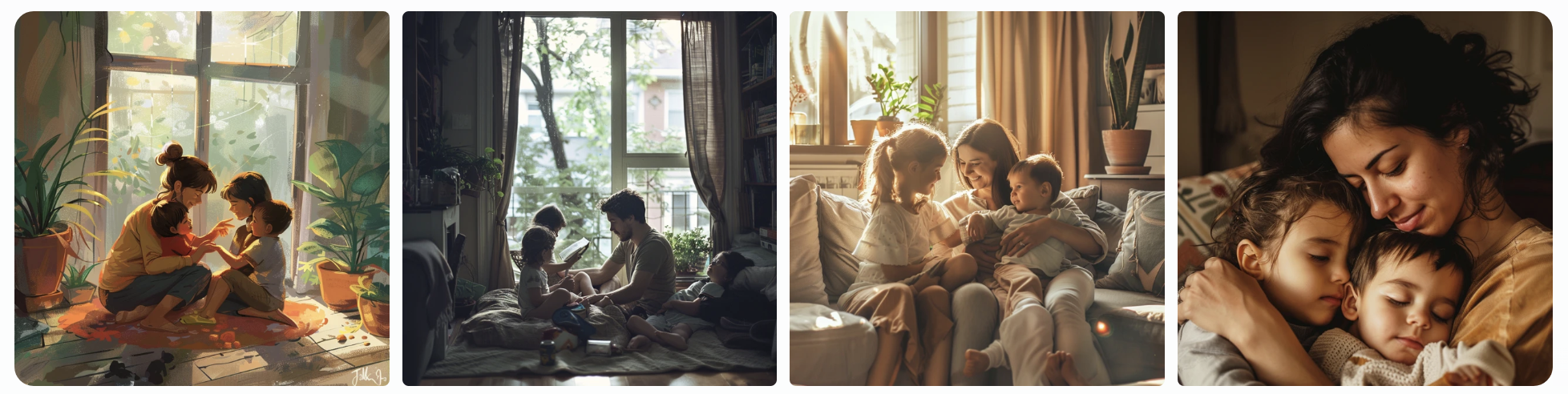
Mitigating Bias
Users can mitigate biases in GenAI models by employing diverse and inclusive language in their prompts to encourage a broader range of outputs. For example:
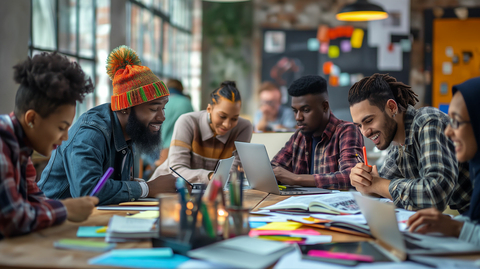
Prompt: black firefighter
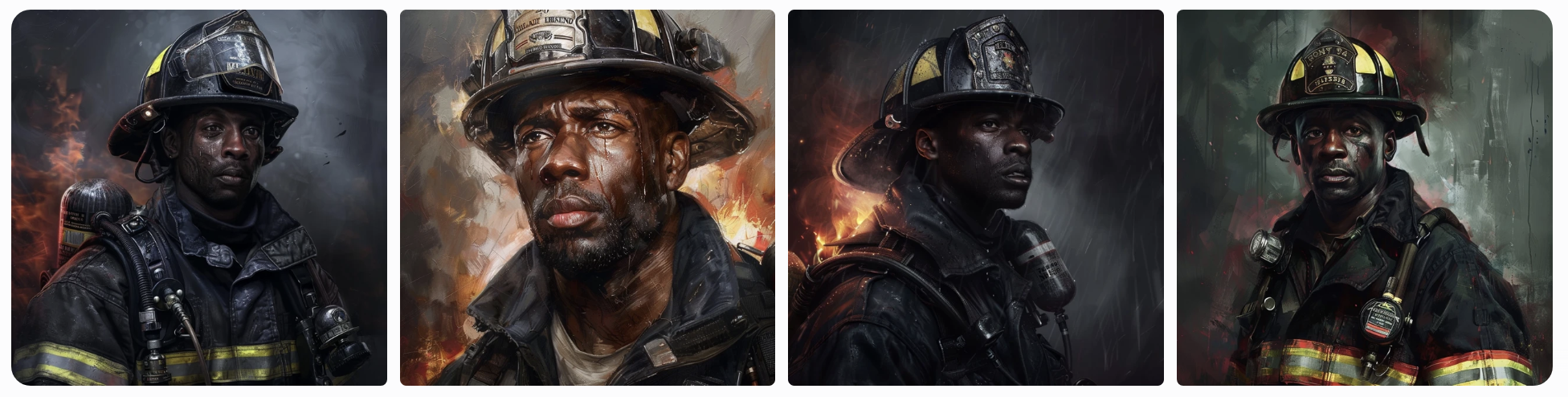
Prompt: female doctor
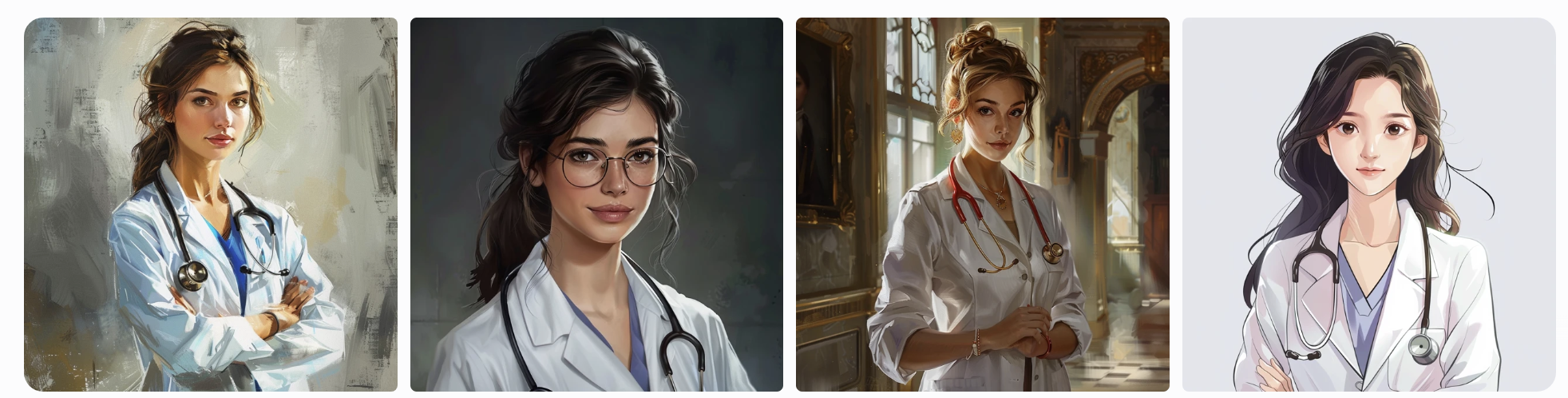
Prompt: Hispanic male nurse
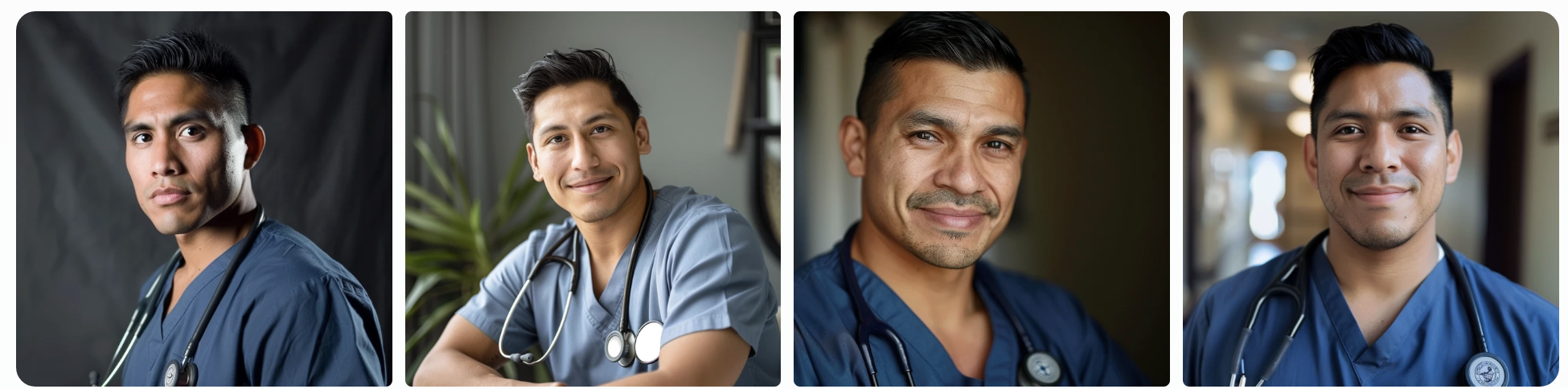
Post-processing generated content to ensure it meets diversity standards and providing feedback to developers can also help improve future versions. Custom training with more diverse datasets and using multiple models to cross-check results can reduce bias. Stay informed about the limitations of GenAI models and educating yourself and others about these issues to foster mindful usage and recognition of biases.